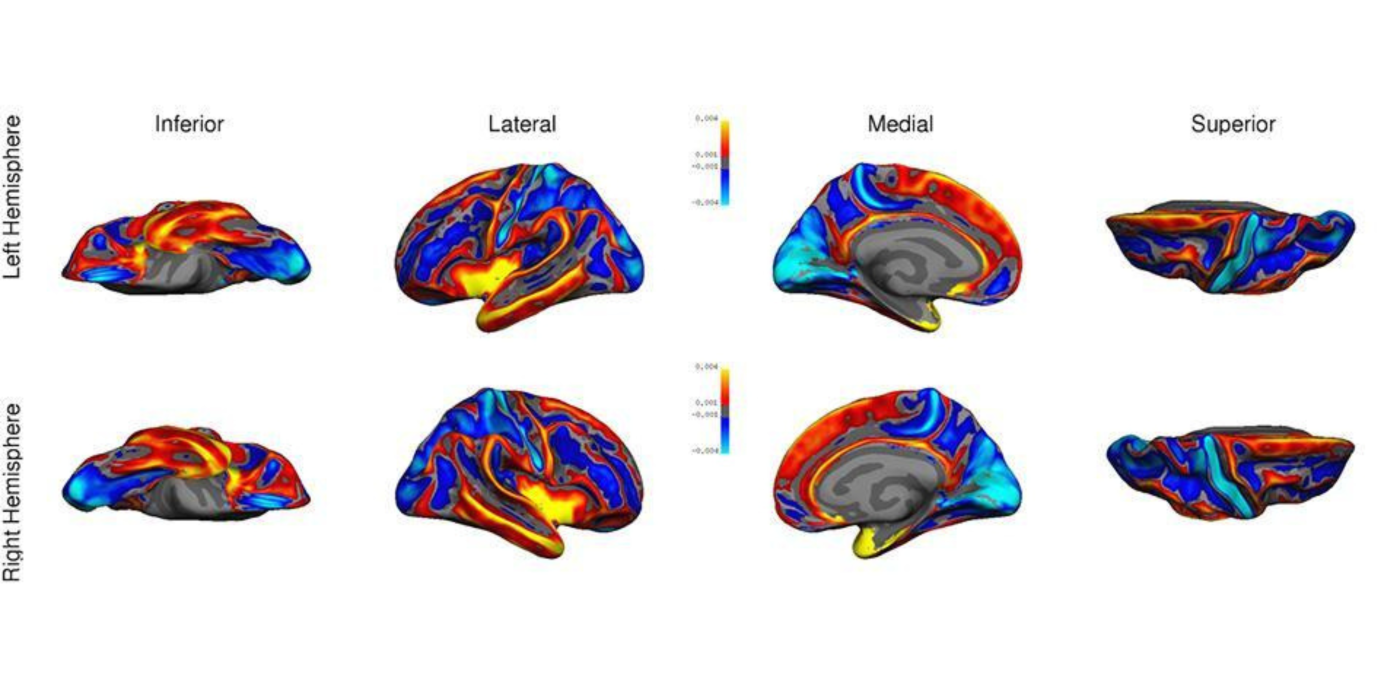
Imaging the hierarchical structure of large scale networks
First principal component of the cortical thickness of 416 healthy participants (20<age<80 years)
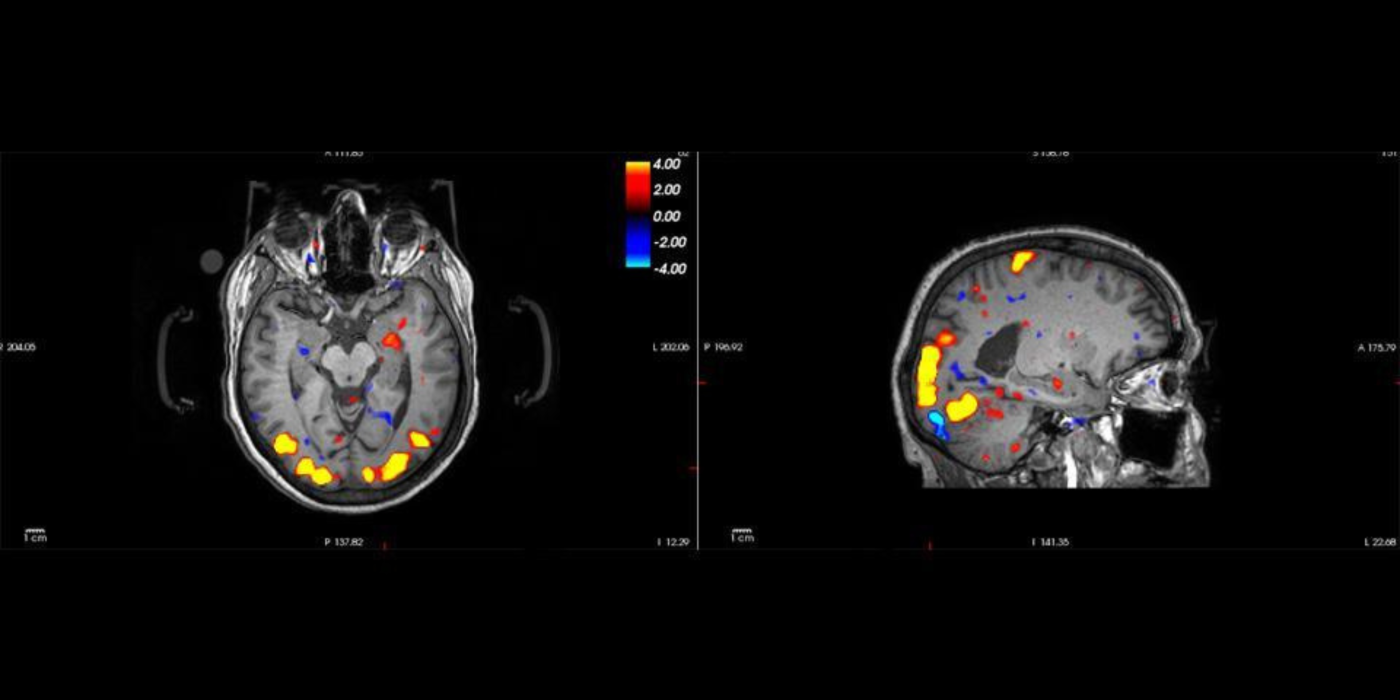
Detecting negative blood oxygen level dependent (BOLD) response in the vicinity of positive BOLD response in the visual cortex due to flashing checkerboard

Quantification of amyloid plaques using in vivo positron emission tomography (PET) scanning with florbetaben on the surface of human brain cerebral cortex and white/gray-matter boundary in a healthy participant
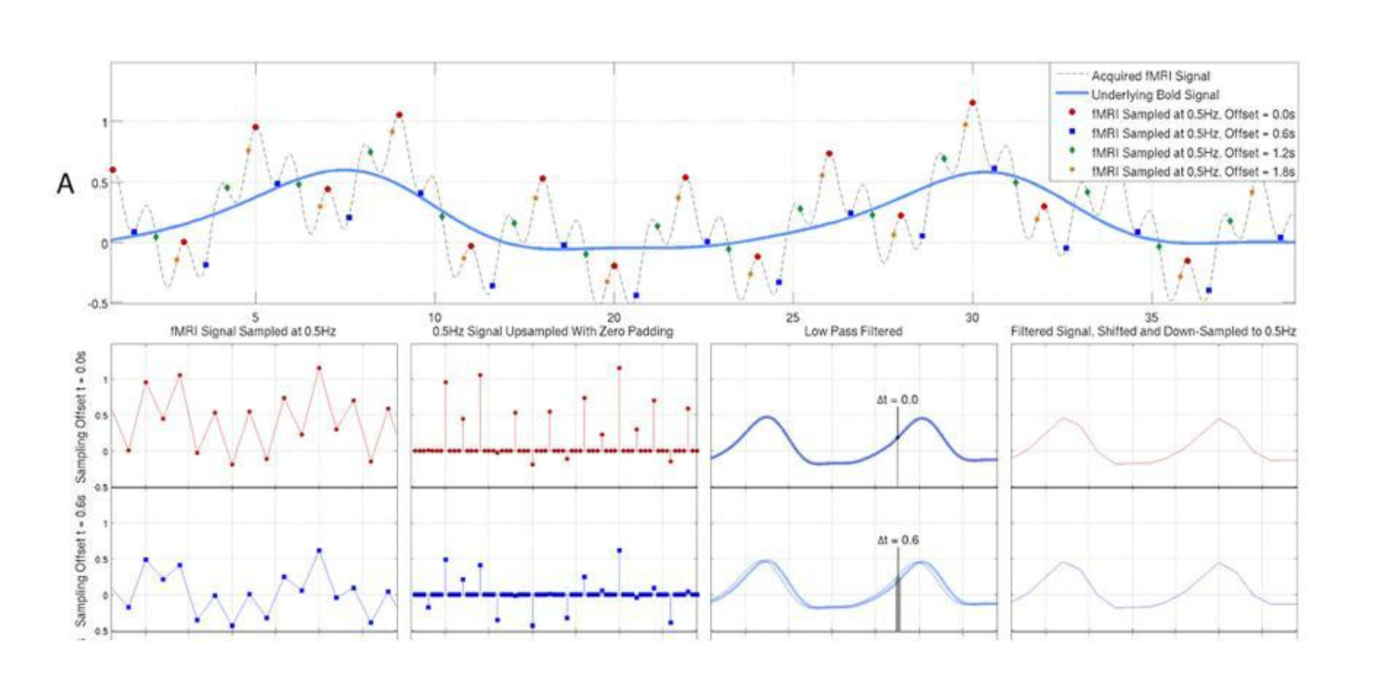
Optimal recovery of blood oxygen level dependent (BOLD) signal
Theoretical illustration of optimal signal reconstruction sampled with different time offset under the Shannon-Nyquist sampling theorem
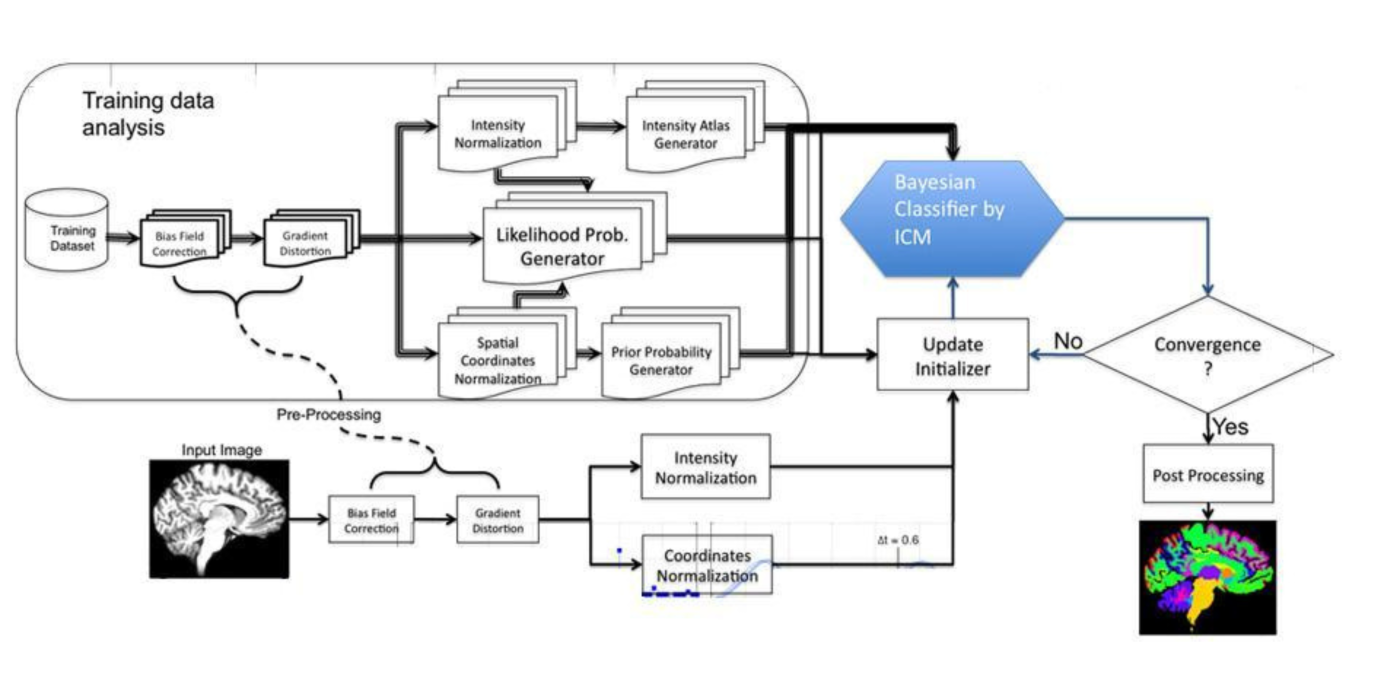
Multilateral Markov random field and brain subcortical segmentation
Flowchart of the pipeline for subcortical segmentation of the human brain using quadrilateral Markov random field and under maximum a posteriori framework

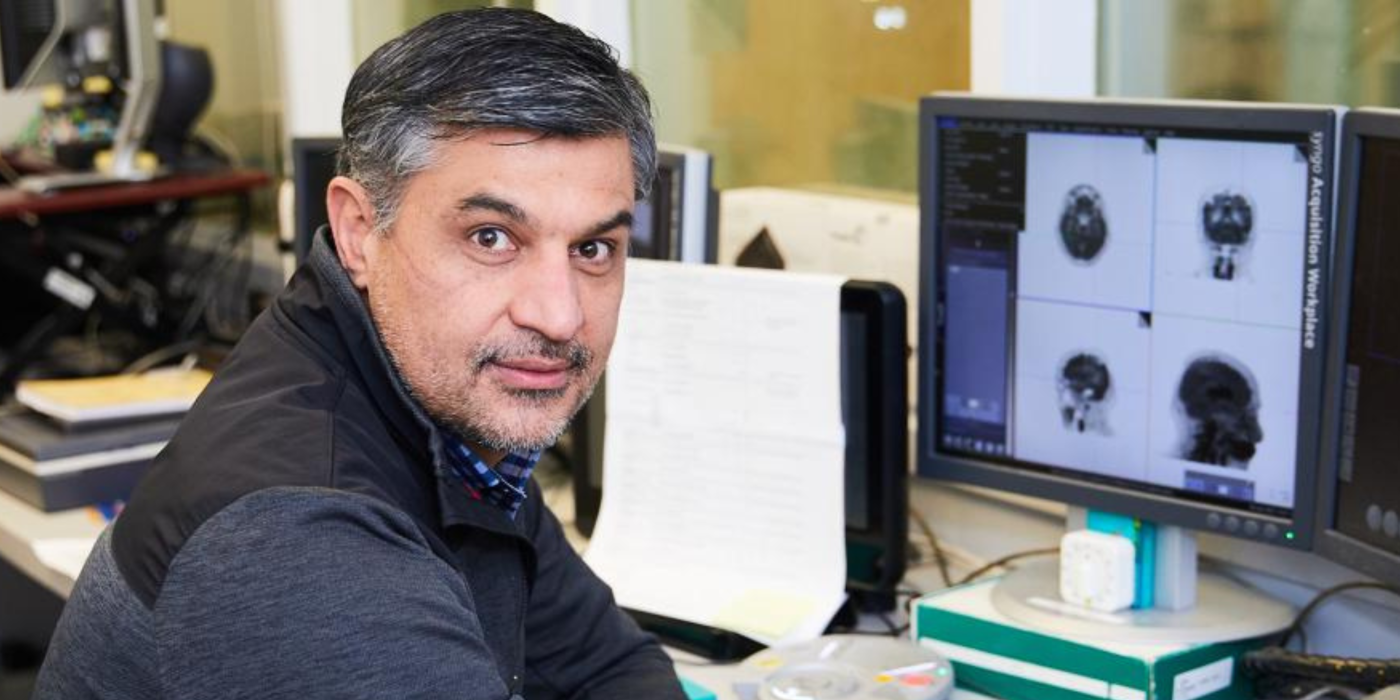